Customer Stories
Use Case: Use AI to lower costs and improve the accuracy of sales predictions for new stores
Background
When deciding on possible new store locations, FamilyMart — a major convenience store chain — tried predicting average daily sales for one year. To do this, they would run a multiple regression analysis based on factors like population, number of parking spots, and the like. However, their predictions would often vary wildly in accuracy.
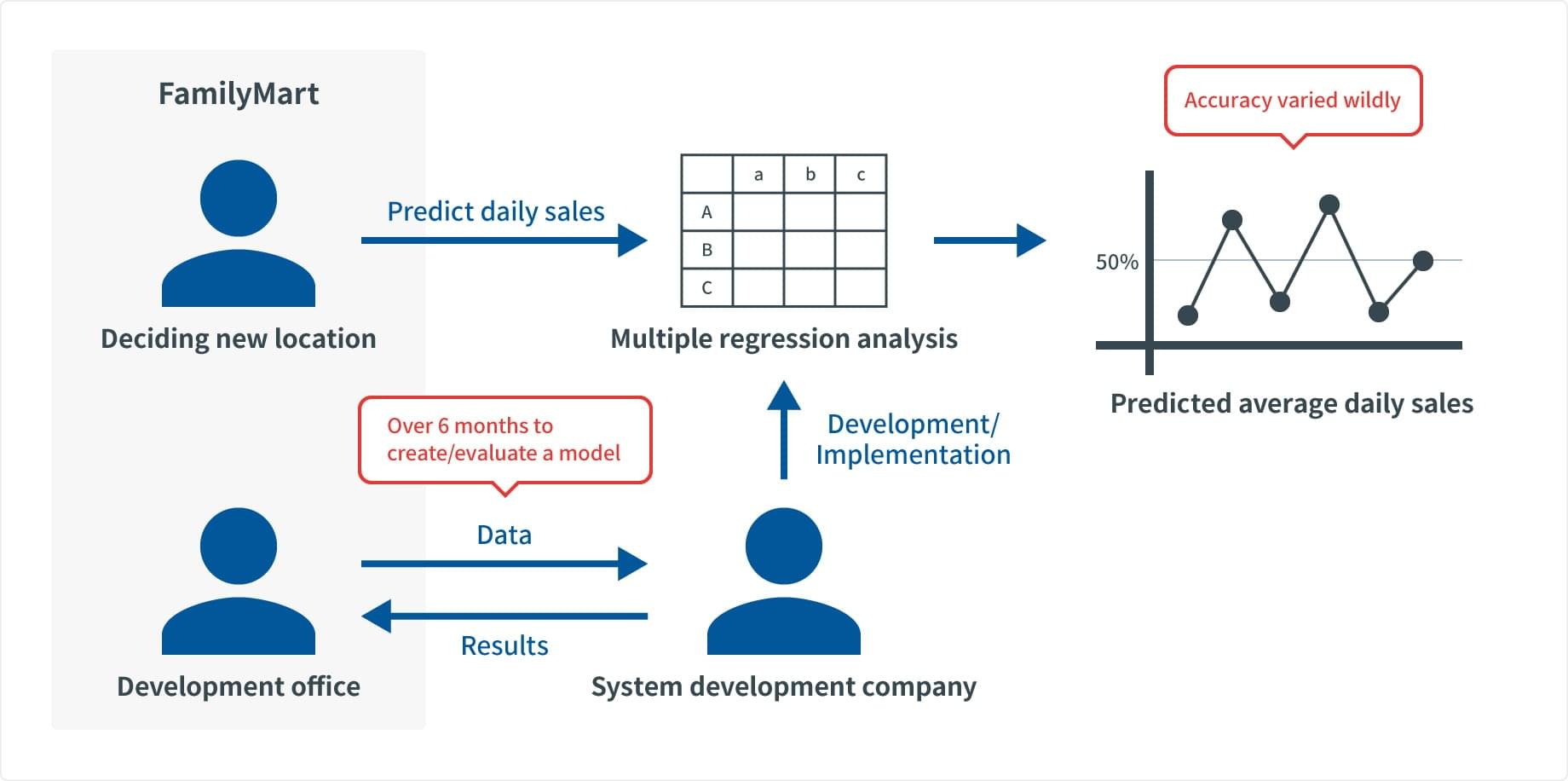
The Solution
FamilyMart started looking for AI solutions to help them get more accurate predictions. They contacted seven companies about building proof of concept models, but most came back with quotes with high initial costs. They instead decided to try the MAGELLAN BLOCKS cloud machine learning service’s regression type Model Generator, which anyone can use from a short term on a flat-rate basis.
With their new MAGELLAN BLOCKS model, the difference between predicted and actual sales was within about $500 for approximately 60% of stores — much better than their previous results. They could also run the system three times more quickly, since they could use MAGELLAN BLOCKS to train their own model with their data. And in terms of cost, MAGELLAN BLOCKS cost them less than half of what they were paying for their previous multiple regression analysis systems. FamilyMart is now making plans to use AI with MAGELLAN BLOCKS to automatically evaluate new store locations.
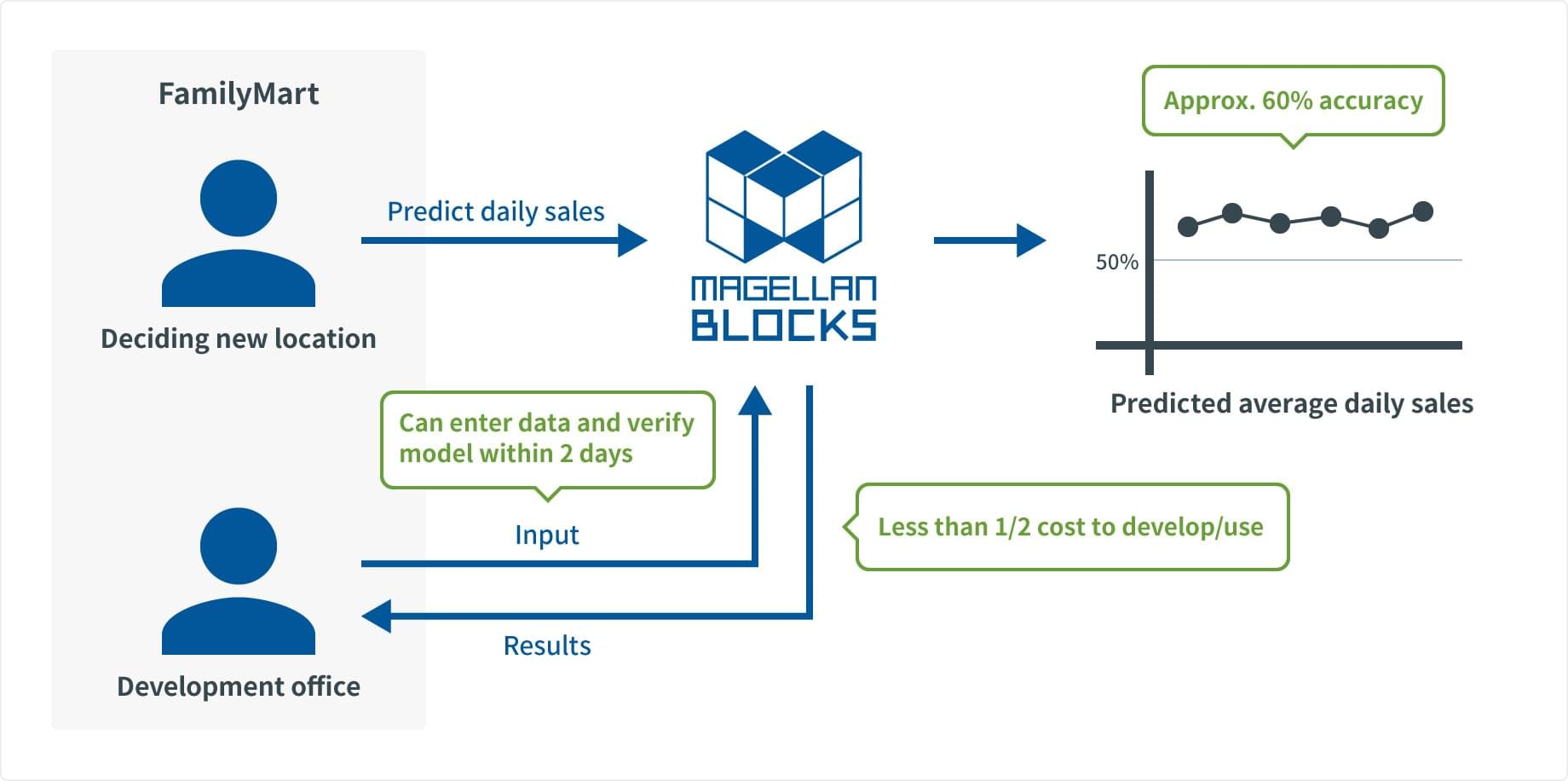